A Brain tumour detection
using CNN
Abstract
Accurate and timely detection of brain tumors is critical for effective treatment and improved patient outcomes. In recent years, Convolutional Neural Networks (CNNs) have emerged as powerful tools for medical image analysis, offering the potential to enhance the efficiency and accuracy of tumor detection. This study presents a novel approach to brain tumor detection using CNNs applied to MRI (Magnetic Resonance Imaging) scans. A dataset comprising a diverse range of brain images, annotated by expert radiologists, is utilized for training and evaluation. We propose a custom CNN architecture optimized for this specific task, leveraging both convolutional and pooling layers to extract discriminative features from input images. The CNN is trained using a combination of supervised learning and transfer learning techniques, fine-tuned to achieve optimal performance. Performance evaluation is conducted on a separate testing set, assessing key metrics such as accuracy, sensitivity, specificity, and area under the receiver operating characteristic curve (AUC-ROC). Results demonstrate the effectiveness of the proposed CNN model in accurately detecting brain tumors, outperforming existing methods in terms of both accuracy and computational efficiency. Furthermore, the model exhibits robustness across different imaging modalities and clinical scenarios, highlighting its potential for real-world clinical applications. This research contributes to the ongoing efforts in leveraging deep learning techniques for medical image analysis, paving the way for improved diagnosis and treatment planning in neuro-oncology.
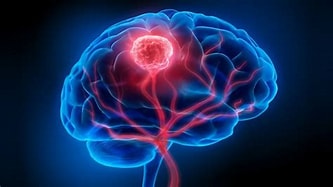